Why do we do data?
What do you actually do with data besides analyzing it and ML/AI?
Mary MacCarthy
September 9, 2022
6 minutes
I recently tweeted out a thought I’d been having about the data space–expressing some doubts about the impact of the work we do:
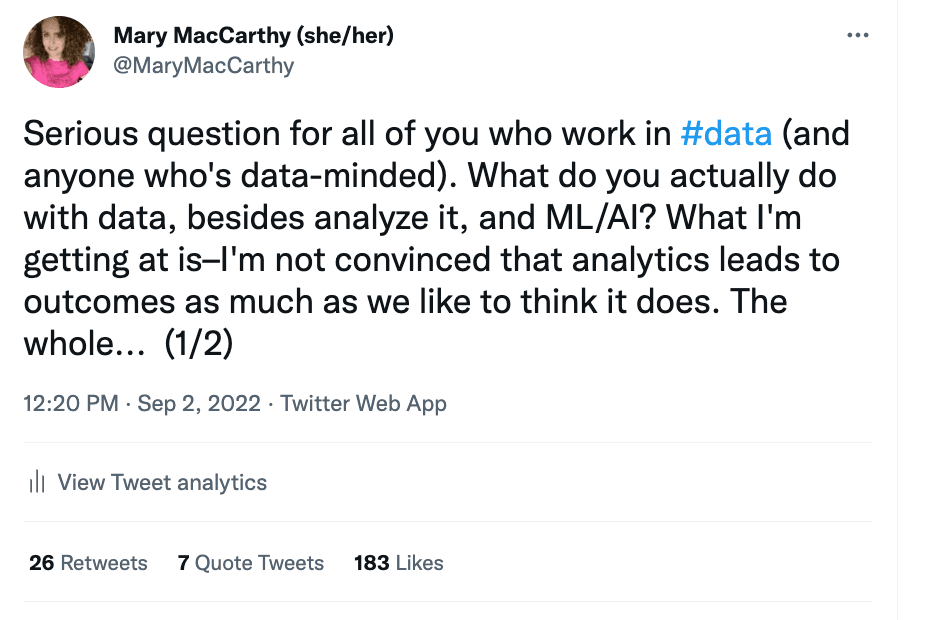
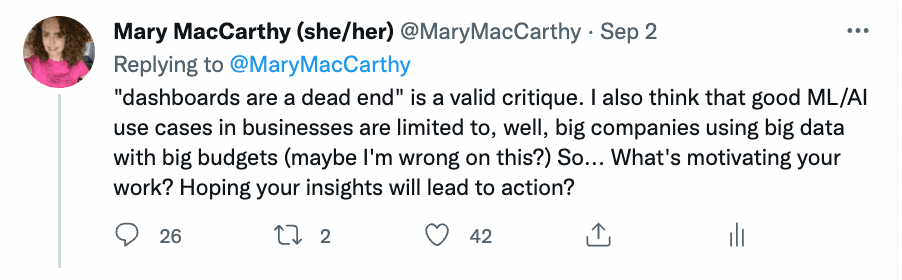
I had hesitated for a while before posting it because I feared responses along the lines of DOES SHE REALLY NOT GET IT? WE DO VERY IMPORTANT WORK, WHICH IS OBVIOUS TO EVERYONE.
Fortunately, as I suspected, many people are asking themselves the exact questions that I voiced.
Also, lots of people aren’t! And they jumped in to defend the work of data teams–providing a multitude of brilliant examples from across industries.
I learned so much.
Below are my thoughts on some of the main themes that emerged in the responses. A huge thank you to everyone who shared their knowledge and opinions; here’s a link to the full thread.
First, The Folks Who Agreed With Me 😂
Lots of people agreed with my prompt that perhaps we’re not doing enough with the data we have, but they each put their own spin on it.
@RealNeilC did not hold back, admitting that the gap between the goals we have for data and the reality of what we do with that data can be huge–even in companies known to be oozing with data talent:
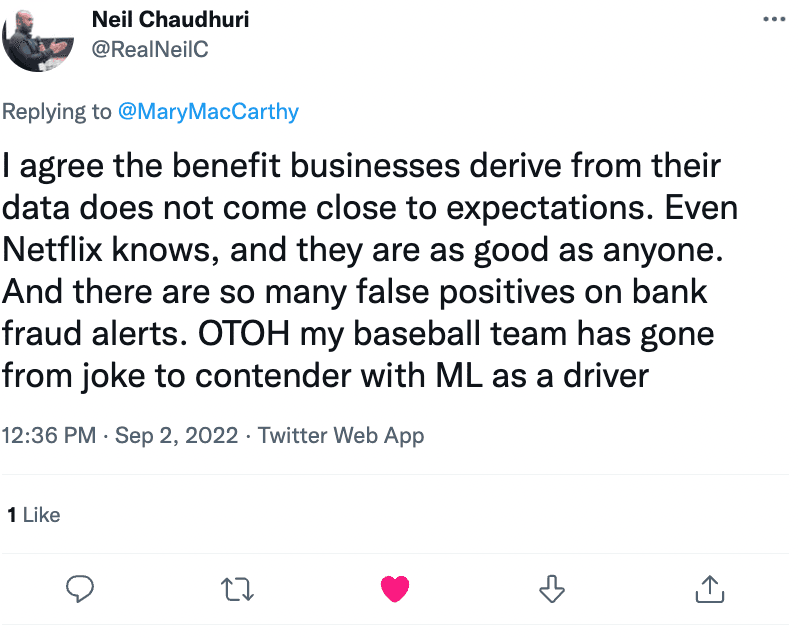
@SalRosario3 suggested that the lack of impact arises from “artificial barriers” that emerge between data teams and business teams–a problem that I see as endemic to contemporary company culture:
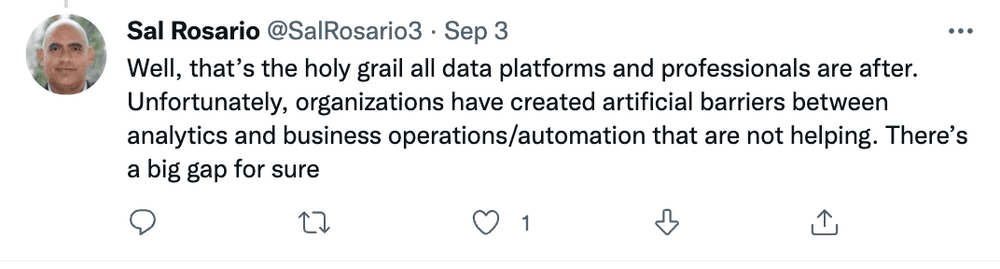
And @Alwaysbmoving praised the value of individual data projects. But he also questioned whether–even in companies where some worthwhile data work is happening–the high-level strategies are actually data-driven.
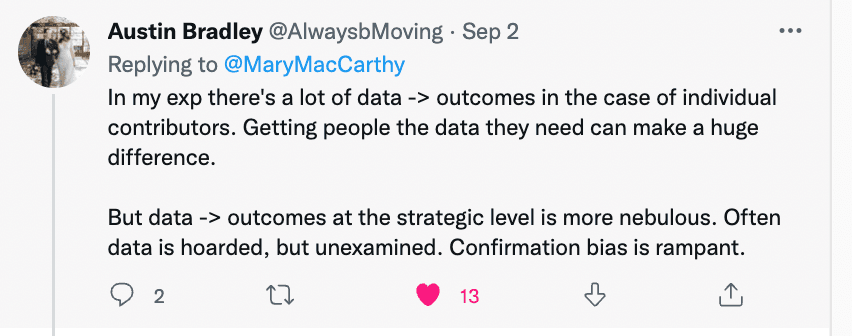
Getting Back to the Basics 🍎
@ChixRivahVA chimed in with a useful reminder that the essence of any good data work is having good data.
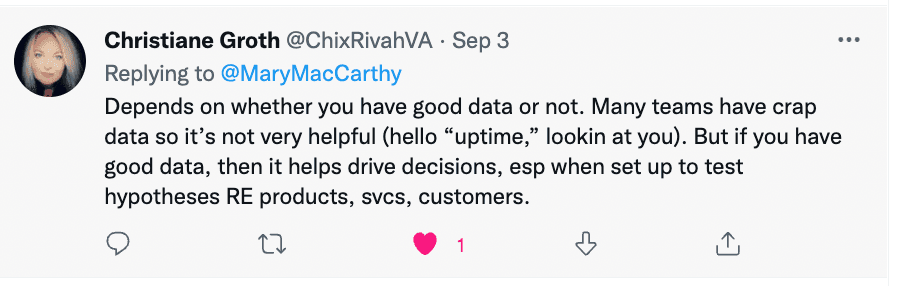
Some of you might say: well, that’s obvious. My response would be: keeping first principles like this in mind is key to ensuring that you’re not wasting time and resources on misguided efforts.
How do you know that your data is good, for the particular question you’re trying to answer or problem you’re trying to solve?
How many of us have the habit of starting our work by asking the question: do I have the best possible data to work with, and if not, how can I obtain it?
If you’re certain that the answer to that question is YES, then you’ll have greater confidence that your insights can eventually help drive decisions in your organization. On that note, I really appreciated @Gdomnenko’s approach–highlighting the effort that goes into getting and cleaning the right data for a particular problem:
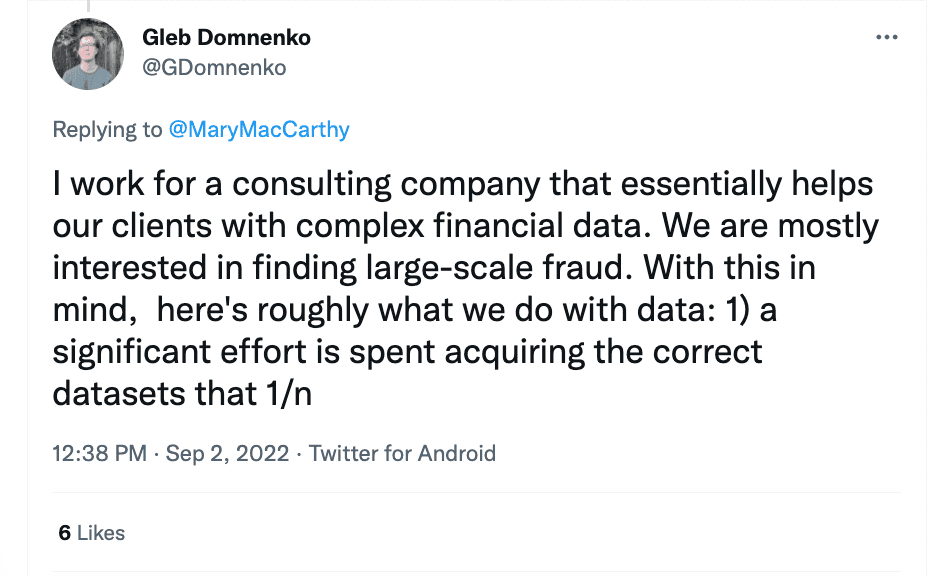
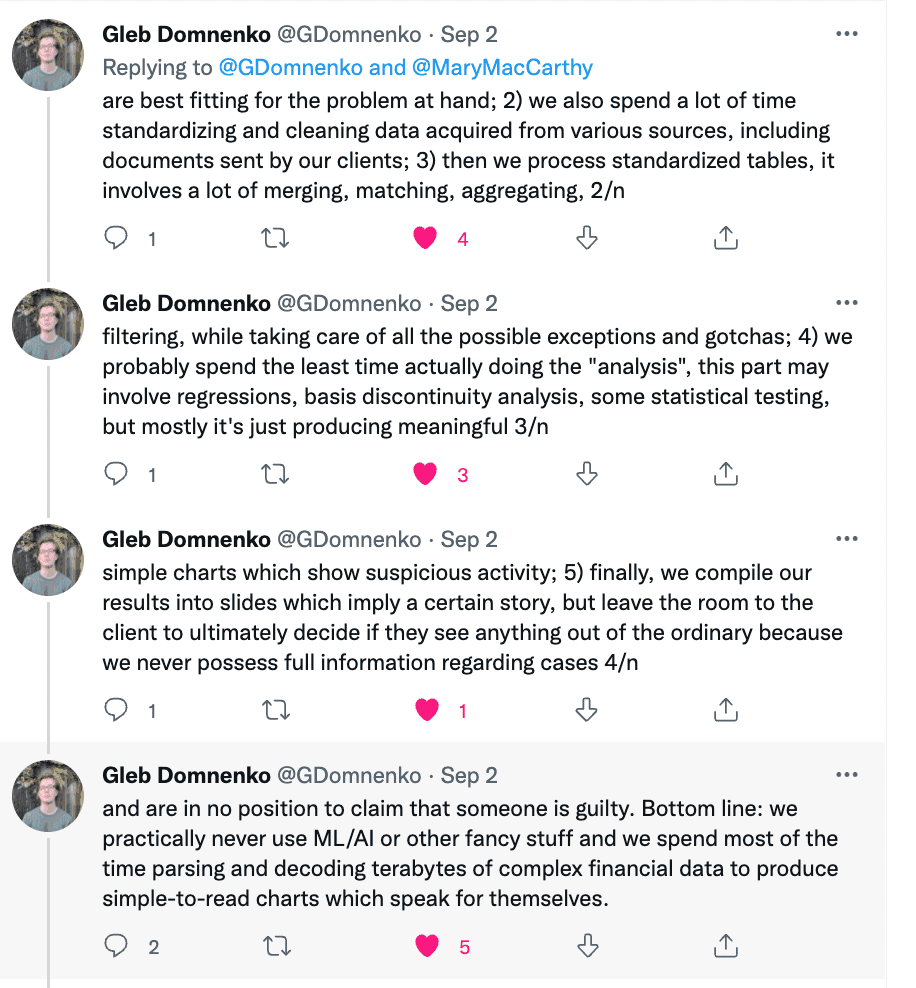
Data Work to Improve Organizational Culture And Happiness 🤓
Some of the strongest messages defending data teams came from people who do people analytics or similar work: gathering and studying data about employees in their own company, and making suggestions for specific organizational actions based on their insights:
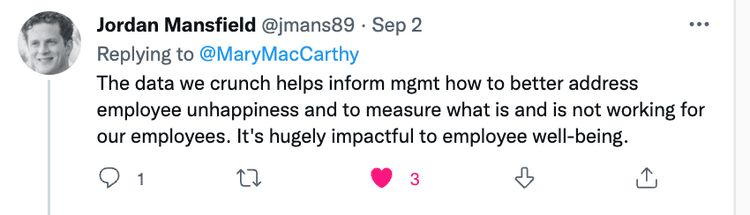
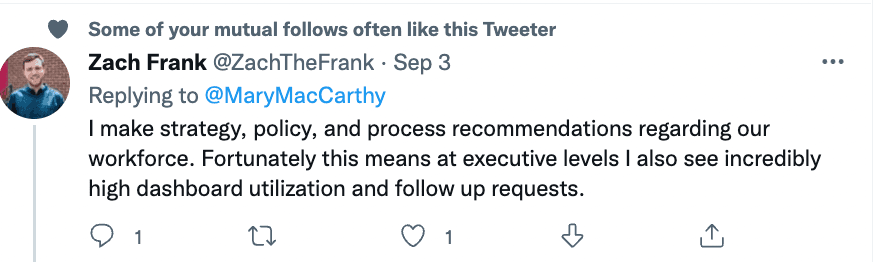
These data practitioners are in a unique position compared to most of us: in people analytics, the data gathering ➡️ analytics ➡️ action timeline can be relatively short, and the area of impact is often visible. If you’re measuring employee happiness metrics, and your colleagues start to seem happier–that must be very satisfying. It kinda makes me want to work in people analytics 🤔.
Machine Learning 💻
A number of voices kindly suggested that my view of ML/AI is nearsighted. I had asked whether machine learning is perhaps needed only in large companies working with very Big Data.
@machsci responded with examples where machine learning algorithms can be applied to specific business problems, even in small organizations–ranging from marketing campaigns to supply chain management, to simply making sure that a company is actually using all the data it has collected:
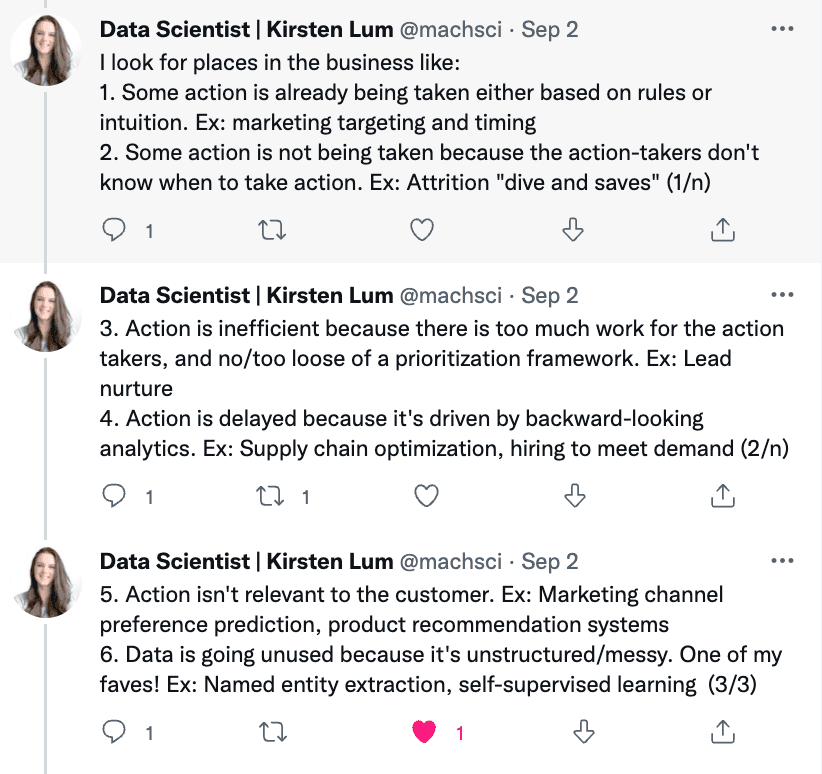
@machsci also had terrific insights into how the work of data teams can be held back by ineffective communication and an inability to influence leadership. I’d suggest reading her entire thread and giving her a follow.
Experiments 📈📉
A number of you pointed out a use case of data that I tend to overlook: experiments:
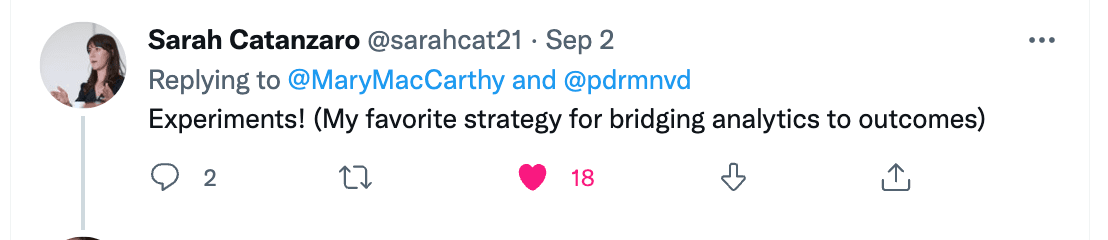
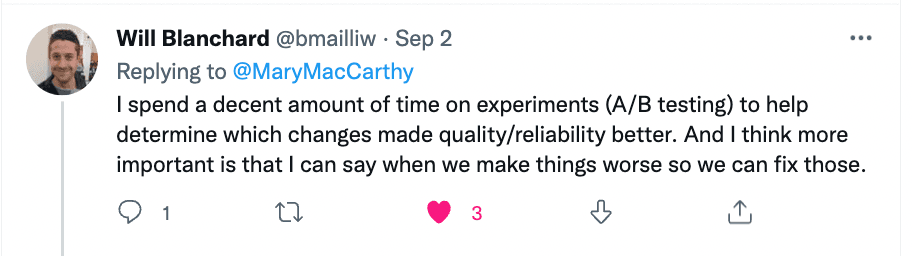
In my defense, I tend to forget about experimentation because in many organizations (including the ones I’ve worked for) it’s not part of the workflow. If you’re like me and you’re inspired to learn more about data experiments, I’d recommend starting with this recent thread by data brain @pdrmnvd.
Data Activation⚡
Some of you made suggestions that very much align with my own assessment of what’s missing from many data workflows: the fact we shouldn’t be stopping at analytics, but should also be taking action on our data (e.g., automatically sending it to business teams, who can use it to drive their day-to-day actions):
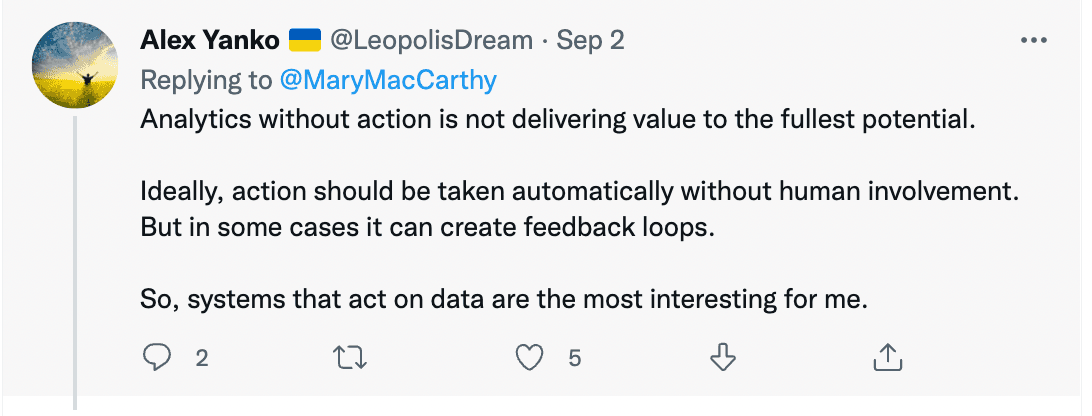
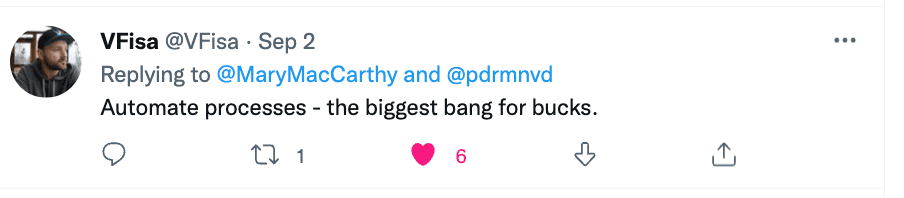
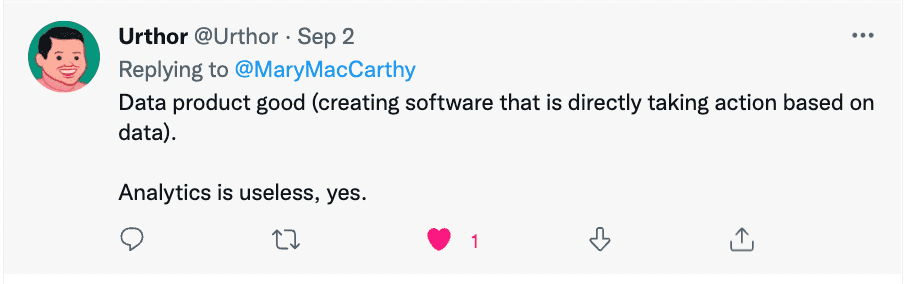
My absolute favorite response came from @AmandiNancy. (It’s my favorite not just because her vision of Data Activation aligns with my own 🤣, but also because she so eloquently explains why operationalizing data is so important).
@AmandiNancy’s initial response to my Tweet was that we can do more with data by improving our storytelling about data. Which is a valid point, and a skill that most data practitioners do need to improve.
But she followed the Twitter discussion closely, and came around to also embracing the need to take action on data, describing her thought process in her own thread.
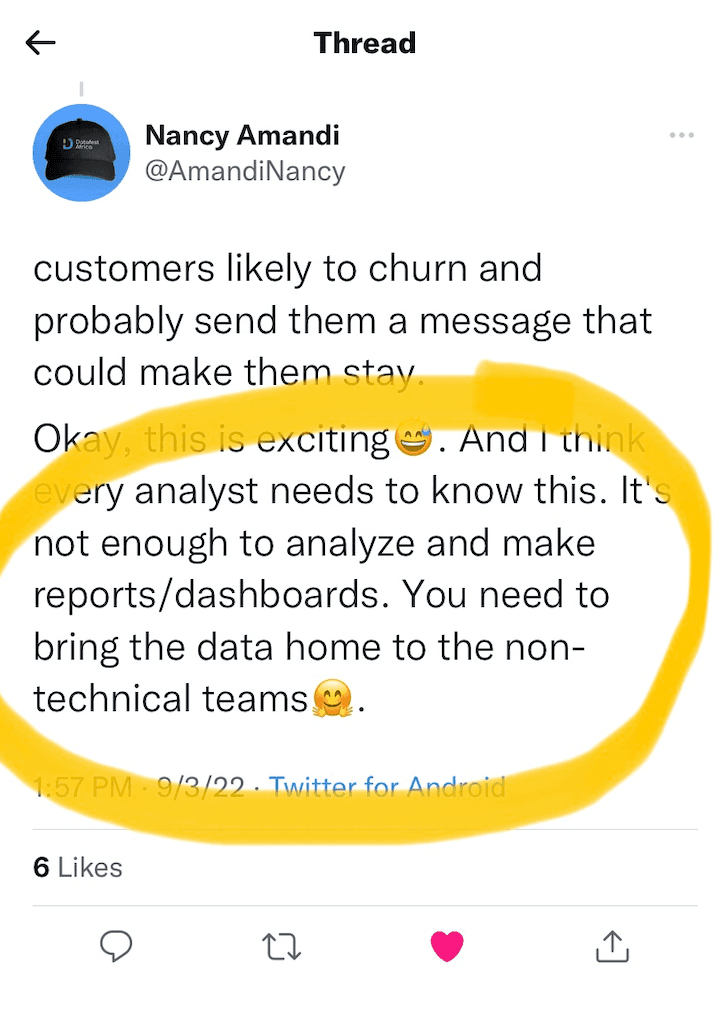
Data Work For The Public Good 💜
For most of us, the work we do every day is motivated by our employer’s mission to make money. That’s not a bad thing, as long as the core of the work is ethical.
But, gosh, it was so nice to hear from data practitioners whose work is about more than just maximizing profit. That includes @bluonvue who wrote about her work analyzing K-12 education data, @IsabelAphrael who has broad experience in public policy data, and public health physician @VarugheseDeepak:
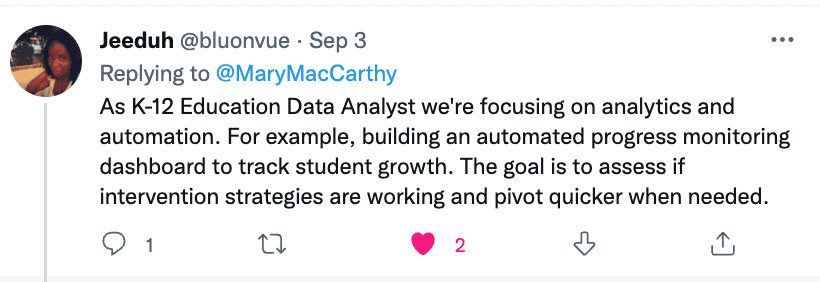
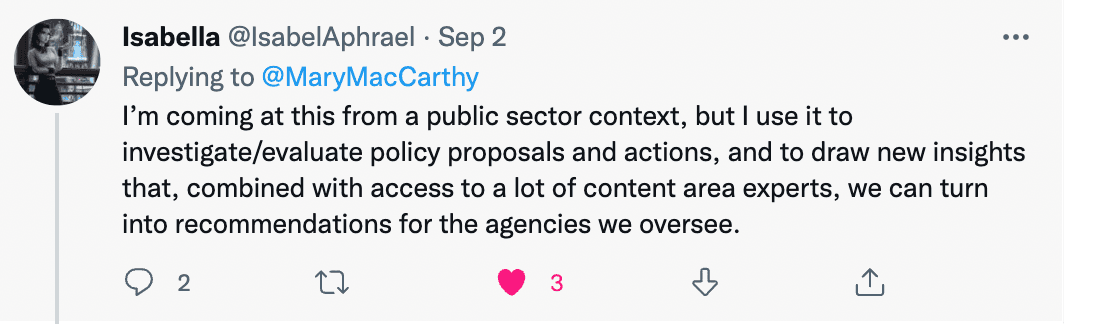
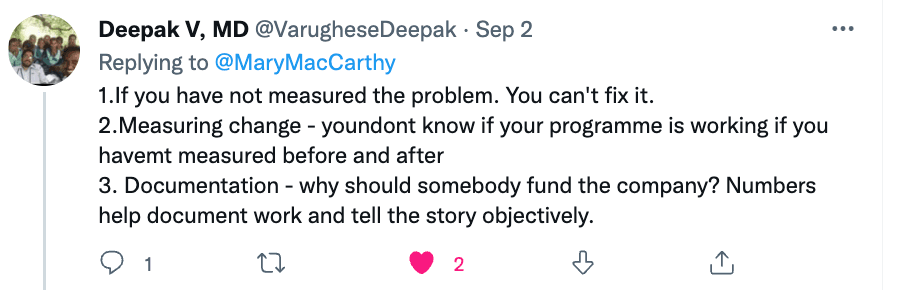
I’m not suggesting that we all should aim to work for nonprofits or government agencies; their work can sometimes be as ethically problematic as anything in the private for-profit sphere. But it sure is good to be reminded that data work can be led by altruistic goals, sometimes with direct & meaningful impacts on human beings.
I love the idea of greater interaction between data teams in the private for-profit world and people like @jeeduh, @IsabelAphrael, and @VarugheseDeepak. Let’s keep these conversations going!!
Mary MacCarthy is a Data Advocate at Hightouch. You can find her at mary@hightouch.com or on Twitter @MaryMacCarthy.